Enhancing Retail Efficiency with Cutting-Edge AI and Machine Learning Analytics Solutions
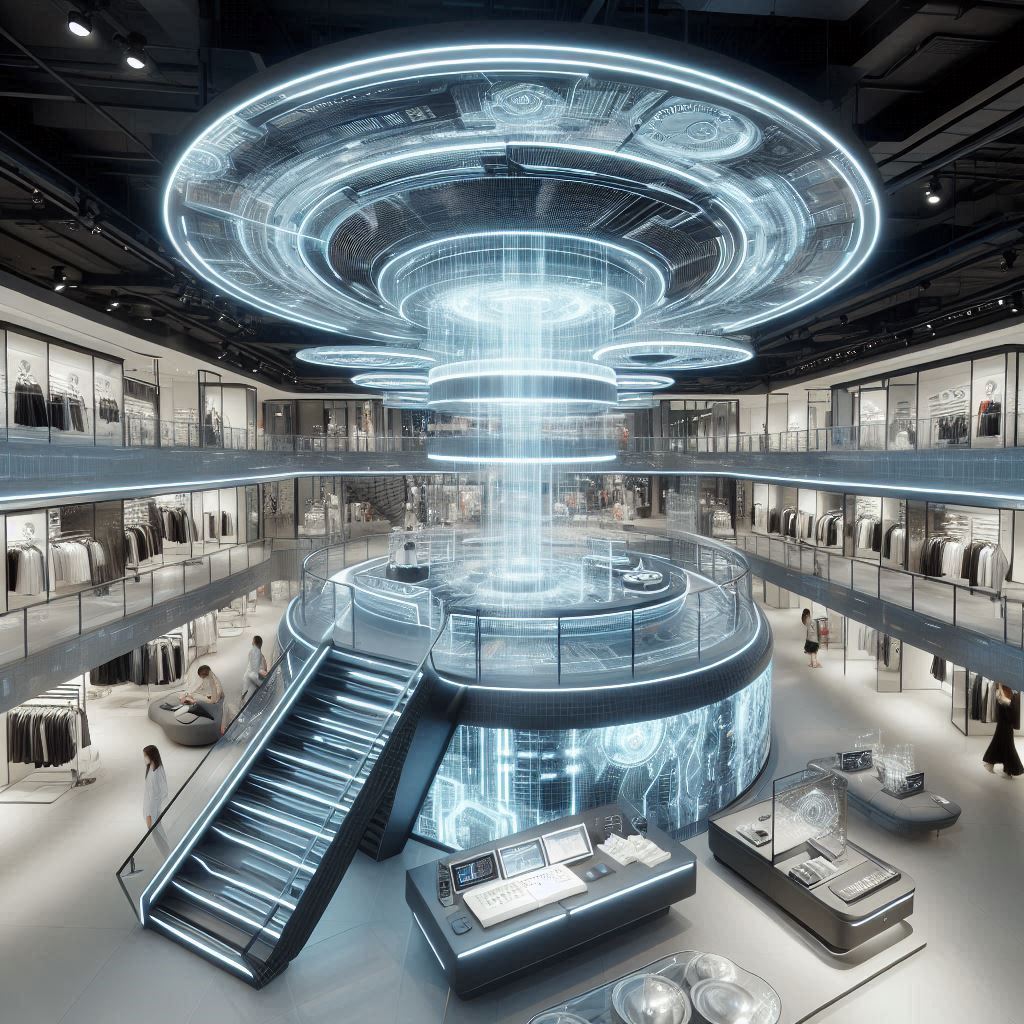
In the intensely competitive retail environment, the integration of advanced data analytics has emerged as a crucial strategy for optimizing performance and achieving superior outcomes. Retailers are progressively utilizing data-driven insights, powered by machine learning and artificial intelligence, to refine customer engagement, enhance operational efficiencies, and boost profitability. This in-depth guide examines the challenges and opportunities inherent in deploying advanced data analytics within the retail sector, exploring various data modalities, essential analytical methodologies, and the transformative potential of AI-driven insights. It further details the practical applications and strategic advantages of leveraging sophisticated analytics tools to drive innovation and competitive differentiation in retail operations.
Advanced-Data Analytics in Retail
The adoption of advanced data analytics in the retail sector involves navigating substantial challenges while also uncovering substantial opportunities. Mastery of these complexities enables retailers to fully harness the potential of their data assets. Leveraging AI and machine learning methodologies can significantly enhance data interpretation and application, driving transformative outcomes and strategic advancements in retail operations.
Challenges:
- Data Integration: Integrating disparate data sources, such as point-of-sale (POS) systems, e-commerce transactions, and customer loyalty programs, presents a complex challenge. Ensuring that this data is synchronized, accurate, and readily accessible demands robust data management frameworks and advanced integration solutions.
- Data Quality: The integrity of analytical outcomes hinges on the quality of the data. Inaccurate or inconsistent data can undermine the reliability of insights. Implementing stringent data validation and cleansing protocols is critical to maintaining high data quality standards.
- Privacy Concerns: As the use of advanced analytics expands, compliance with data protection regulations such as GDPR and CCPA becomes imperative. Striking a balance between comprehensive data collection and safeguarding customer privacy is essential for regulatory adherence and maintaining consumer trust.
- Technological Barriers: Deploying sophisticated analytics solutions necessitates considerable investment in cutting-edge technologies and specialized talent. Smaller retailers, in particular, may face challenges related to the financial and technical demands associated with advanced analytics infrastructure.
Opportunities:
- Personalised Customer Experiences: Leveraging customer data through advanced analytics enables retailers to deliver highly personalized recommendations, precision-targeted promotions, and enhanced customer service, thereby increasing customer satisfaction and fostering long-term loyalty.
- Inventory Optimization: Utilizing predictive analytics and machine learning algorithms allows retailers to accurately forecast demand patterns, thereby optimizing inventory levels. This approach mitigates the risks of stockouts and overstock situations, leading to cost efficiencies and revenue growth.
- Price Optimization: Implementing dynamic pricing models driven by data analytics facilitates real-time adjustments to pricing strategies based on variables such as demand fluctuations, competitive pricing, and market conditions, thus maximizing profitability.
- Enhanced Marketing: Data-driven marketing analytics empower retailers to refine campaign strategies by targeting specific customer segments with tailored messaging at optimal times, thereby significantly improving campaign effectiveness and return on investment.
Data Challenges
Data serves as the foundation for any sophisticated analytics strategy. Nevertheless, the effective management and utilization of data involve a distinct set of complexities. Addressing these challenges is crucial for optimizing the value derived from data-driven insights.
Volume, Variety, and Velocity:
- Volume: Retailers accumulate extensive datasets from numerous sources, necessitating advanced data storage and processing infrastructure to manage this high volume effectively.
- Variety: Retail data encompasses a range of formats, including structured data (e.g., sales transactions), semi-structured data (e.g., social media interactions), and unstructured data (e.g., customer reviews). Integrating and analysing these heterogeneous data types presents significant challenges.
- Velocity: The dynamic nature of retail operations demands real-time data ingestion and analysis. Ensuring data currency and timely delivery of actionable insights is critical for informed decision-making and operational agility.
Data Silos:
Data silos emerge when information is compartmentalized across disparate departments or systems, impeding a unified perspective of business operations. Mitigating these silos through the implementation of integrated data platforms enables the consolidation of data, facilitating a holistic analysis and delivering comprehensive insights.
Data Governance:
Implementing robust data governance frameworks is essential for ensuring data integrity, security, and regulatory compliance. This encompasses the establishment of clear data stewardship roles, the application of stringent data standards, and the continuous oversight of data utilization practices.
Types of Data
Grasping the various data types prevalent in retail is crucial for formulating effective analytics strategies. Recognizing these data modalities is fundamental to leveraging advanced analytics techniques and deriving actionable insights.
Customer Data:
- Demographic Data: Information on customer attributes such as age, gender, income, and geographic location facilitates effective segmentation and targeting strategies.
- Behavioural Data: This includes comprehensive records of customer interactions, such as purchasing history and online browsing behaviours, which are essential for data-driven decision-making and optimizing marketing strategies.
- Feedback and Reviews: Customer reviews and feedback offer valuable qualitative insights into consumer preferences and pain points, informing product development and service improvements.
Sales Data:
- Transaction Data: Detailed logs of sales transactions, encompassing items purchased, pricing information, and payment methods, are crucial for analyzing sales performance and consumer purchasing patterns.
- POS Data: Data extracted from point-of-sale (POS) systems, which captures in-store sales transactions and customer interactions, is vital for operational analysis and inventory management.
Operational Data:
- Inventory Data: Metrics on stock levels, inventory turnover rates, and replenishment cycles are essential for managing inventory efficiently and optimizing supply chain operations.
- Supply Chain Data: Information regarding supplier performance, logistics operations, and delivery timelines is critical for enhancing supply chain effectiveness and ensuring timely product availability.
Marketing Data:
- Campaign Data: Metrics derived from marketing initiatives, such as click-through rates and conversion rates, are essential for assessing campaign effectiveness and optimizing strategies.
- Social Media Data: Analytical insights from social media interactions, mentions, and sentiment analysis provide critical information on brand perception and customer engagement dynamics.
Advanced Retail Analytics
Advanced retail analytics harnesses sophisticated methodologies and technologies to derive actionable insights from data. Key approaches include:
- Descriptive Analytics: This approach focuses on the aggregation and summarization of historical data to elucidate past events. Techniques include:
- Data Aggregation: Integrating data from diverse sources to enable comprehensive and unified analysis.
- Data Visualization: Employing visual representations such as charts, graphs, and dashboards to facilitate data interpretation and insight communication.
- Predictive Analytics: Utilizing historical data to project future trends and outcomes. Techniques include:
- Regression Analysis: Analysing relationships between variables to forecast potential future results.
- Machine Learning: Deploying algorithms that continuously learn from data to enhance prediction accuracy.
- Prescriptive Analytics: Providing actionable recommendations based on predictive insights. Techniques include:
- Optimization Models: Utilizing mathematical frameworks to identify optimal decision-making paths.
- Simulation: Creating and analysing scenarios to evaluate the potential impacts of different strategies.
- Diagnostic Analytics: Investigating data to uncover the underlying causes of past performance. Techniques include:
- Root Cause Analysis: Identifying the fundamental factors contributing to performance issues.
- Correlation Analysis: Assessing the relationships between various data points to understand their influence on outcomes.
Unveiling the Power of Retail Data Analytics
Retail data analytics encompasses various methodologies, each providing distinct insights and advantages. Key approaches include:
Customer Analytics:
Customer analytics aims to decipher consumer behaviour and preferences to enhance the shopping experience. Core techniques involve:
- Segmentation: Categorizing customers based on demographic attributes to enable targeted marketing and product development strategies tailored to distinct consumer segments.
- Churn Analysis: Employing predictive models to identify customers at risk of discontinuing their engagement and implementing targeted retention initiatives to mitigate attrition.
- Lifetime Value Analysis: Utilizing predictive analytics to estimate the long-term economic value of customers, thereby allowing businesses to focus on and prioritize high-value customer segments.
Sales and Market Basket Analysis:
Sales and market basket analysis utilizes advanced analytics to scrutinize sales patterns and product associations. Key techniques include:
- Market Basket Analysis: Employing association rule mining and transactional data analysis to identify frequently co-purchased products, thereby enhancing cross-selling and upselling strategies.
- Sales Trend Analysis: Applying time series analysis and predictive modelling to monitor sales trends, informing inventory management and marketing strategy adjustments.
Inventory and Supply Chain Analytics:
Inventory and supply chain analytics focus on optimizing stock levels and logistics operations. Key techniques include:
- Demand Forecasting: Utilizing predictive analytics and machine learning algorithms to forecast future demand, ensuring optimal inventory levels and reducing stockouts or overstock situations.
- Supplier Performance Analysis: Implementing performance metrics and data-driven evaluations to assess supplier reliability and efficiency, enhancing supply chain resilience and performance.
Marketing and Campaign Analytics:
Marketing and campaign analytics assess the effectiveness of marketing initiatives through various data-driven approaches. Key techniques include:
- Attribution Modelling: Applying multi-touch attribution and econometric modeling to determine the contribution of various marketing activities to sales outcomes.
- Campaign Performance Analysis: Utilizing performance metrics and analytical techniques to evaluate the success of marketing campaigns, focusing on metrics such as return on investment (ROI) and conversion rates.
What Information Gets Collected in Retail Data Analytics
Retailers aggregate a comprehensive array of data to derive actionable insights into operational efficiency and consumer behavior. This includes:
Customer Information:
- Demographics: Attributes such as age, gender, income, educational background, and geographic location.
- Behavioural Data: Metrics encompassing purchase history, browsing behaviors, and interactions with marketing initiatives.
- Feedback and Reviews: Qualitative assessments and ratings provided by customers regarding products and services.
Transactional Information:
- Sales Transactions: Detailed records of purchase activities, including product specifics, quantities, and payment methods.
- Returns and Exchanges: Data related to product returns and exchanges, including reasons and transaction details.
Operational Information:
- Inventory Levels: Metrics on current stock status, turnover rates, and instances of stockouts.
- Supply Chain Metrics: Data reflecting supplier performance, delivery timelines, and logistics expenditures.
Marketing Information:
- Campaign Data: Performance analytics from marketing campaigns, including email open rates, click-through rates, and social media engagement metrics.
- Customer Segmentation: Classification of customers based on behavioral patterns, demographic characteristics, or preferences for targeted marketing strategies.
Leveraging Retail Data Analytics: Strategic Applications
Retail data analytics offers a broad spectrum of transformative applications, allowing retailers to optimize operations and enhance customer experiences. Key applications include:
- Personalized Marketing: By leveraging customer data analytics, retailers can design and execute highly personalized marketing campaigns tailored to individual preferences. This includes targeted email outreach, bespoke product recommendations, and customized promotions.
- Inventory Management: Advanced analytics facilitates precise inventory management by forecasting demand trends, identifying slow-moving inventory, and mitigating stockout risks. This ensures optimal product availability and enhances inventory turnover.
- Price Optimization: Data-driven pricing strategies enable real-time price adjustments based on demand fluctuations, competitive dynamics, and market conditions. This approach maximizes profitability while maintaining market competitiveness.
- Enhanced Customer Experience: Utilizing insights into customer behavior and preferences allows retailers to refine the shopping experience through personalized recommendations, tailored promotional offers, and improved customer service.
- Supply Chain Optimization: Data analytics supports the enhancement of supply chain efficiency by evaluating supplier performance, reducing logistics costs, and ensuring timely delivery of goods. This improves overall supply chain resilience and effectiveness.
- Fraud Detection: Advanced analytical techniques identify anomalous patterns and deviations in transaction data, aiding in the detection and prevention of fraudulent activities.
- Market Basket Analysis: Employing market basket analysis helps retailers discern product purchase patterns, informing store layout optimization, cross-selling strategies, and promotional tactics.
- Customer Retention: Through churn analysis, retailers can pinpoint at-risk customers and deploy targeted retention strategies to maintain customer loyalty and engagement.
- Sales Forecasting: Accurate sales forecasting, powered by predictive analytics, enables retailers to anticipate future demand, optimize inventory levels, and align marketing efforts with emerging trends.
- Campaign Effectiveness: Analytics evaluates marketing campaign performance by providing insights into engagement metrics and conversion rates, facilitating continuous optimization and more strategic allocation of marketing resources.
Conclusion
Advanced data analytics transforms the retail sector by delivering actionable insights that drive business success. While challenges exist, the potential benefits of data analytics are substantial. Retailers adept at leveraging data can enhance customer experiences, optimize operational efficiency, and gain a competitive advantage. By understanding the types of data available, employing sophisticated analytical techniques, and applying these insights practically, retailers can achieve significant success and excel in the competitive retail landscape.
FAQ
What are the primary challenges retailers face when implementing advanced data analytics solutions?
Retailers encounter several key challenges in implementing advanced data analytics solutions:
- Data Integration: Integrating data from diverse sources, including point-of-sale (POS) systems, e-commerce transactions, and customer loyalty programs, is complex and necessitates advanced systems to ensure data accuracy and consistency.
- Data Quality: Inaccurate or inconsistent data can result in unreliable insights. Ensuring high data quality through rigorous validation and cleansing procedures is critical.
- Privacy Concerns: Adhering to data protection regulations such as GDPR and CCPA is essential. Retailers must balance the need for comprehensive data collection with stringent privacy requirements.
- Technological Barriers: The implementation of advanced analytics demands substantial investment in technology and expertise, which can be particularly challenging for smaller retailers.
What types of data do retailers typically collect for advanced analytics?
Retailers gather a variety of data types for advanced analytics, including:
- Customer Data: This includes demographic details (e.g., age, gender, income, location), behavioural data (e.g., purchase history, browsing behavior), and qualitative feedback (e.g., customer reviews).
- Sales Data: Records of sales transactions (e.g., products purchased, pricing, payment methods) and data from point-of-sale (POS) systems.
- Operational Data: Information on inventory levels, stock turnover rates, and supply chain metrics (e.g., supplier performance, delivery timelines).
- Marketing Data: Metrics related to campaign performance (e.g., click-through rates, conversion rates) and social media engagement analytics.
How can advanced data analytics enhance customer experience in retail?
Advanced data analytics can significantly enhance the customer experience by:
- Personalized Marketing: Analysing customer data allows for the development of targeted marketing campaigns, personalized product recommendations, and tailored promotional offers.
- Improved Customer Service: Insights into customer preferences and behaviors enable retailers to provide more personalized and responsive customer service.
- Enhanced Shopping Experience: Data-driven insights optimize store layouts and online interfaces to align with customer preferences, resulting in a more engaging shopping experience.
What are some practical applications of retail data analytics?
Retail data analytics can be applied in several impactful ways, including:
- Inventory Management: Utilizing predictive analytics to forecast demand and optimize stock levels, thus preventing stockouts and overstock situations.
- Price Optimization: Implementing dynamic pricing models that adjust prices in real-time based on factors such as demand, competitive pressures, and market conditions.
- Market Basket Analysis: Analysing product co-purchase patterns to enhance cross-selling and upselling strategies.
- Sales Forecasting: Employing forecasting models to align inventory management and marketing efforts with anticipated demand.
- Fraud Detection: Applying anomaly detection techniques to transaction data to identify and prevent fraudulent activities by recognizing unusual patterns.
What advanced analytics techniques are commonly used in retail?
Commonly utilized advanced analytics techniques in retail include:
- Descriptive Analytics: Summarizing historical data to understand past performance through data aggregation and visualization methods.
- Predictive Analytics: Using historical data to project future trends and outcomes via regression analysis and machine learning algorithms.
- Prescriptive Analytics: Offering actionable recommendations based on predictive insights using optimization models and simulation techniques.
- Diagnostic Analytics: Investigating data to identify the root causes of performance issues through root cause analysis and correlation assessments.