Optimizing Retail Business Opportunities in 2024 with Advanced Analytics Solutions
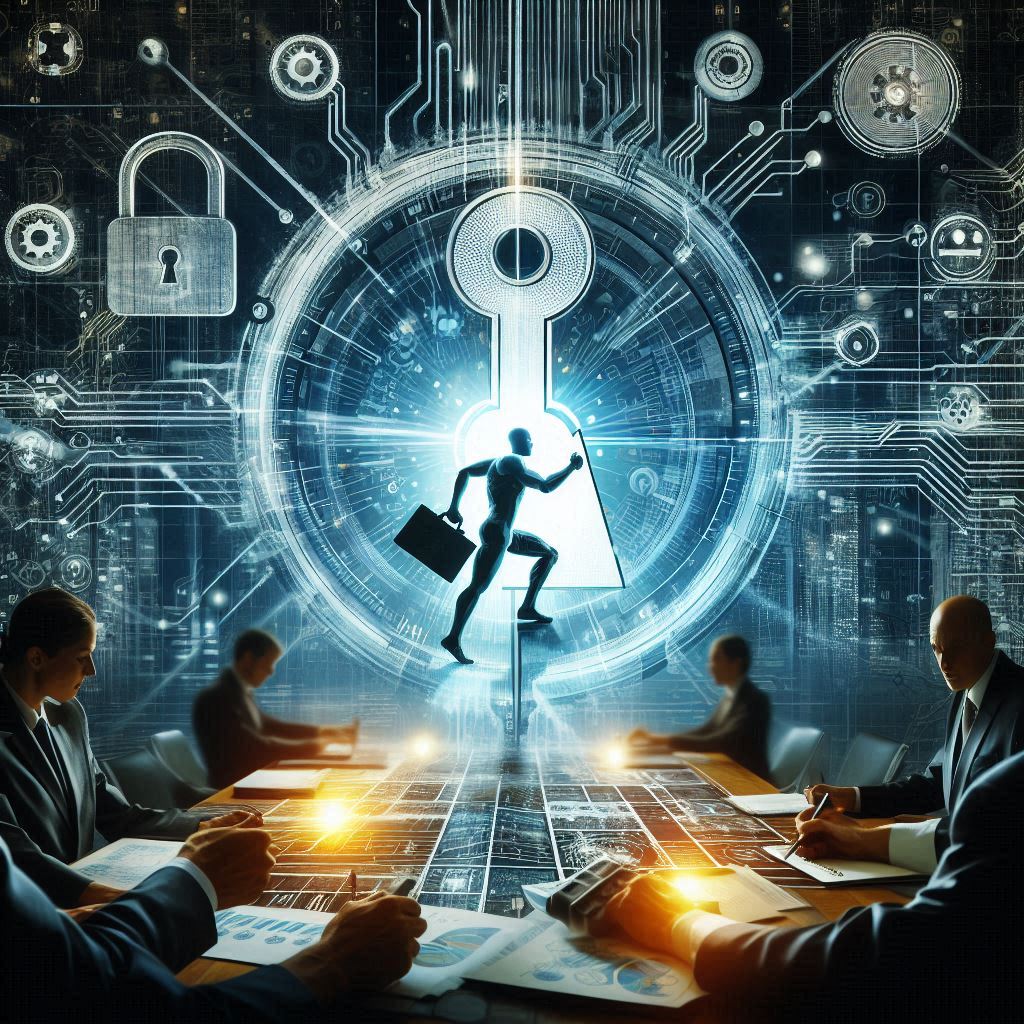
Introduction
In the dynamic retail landscape, understanding customer behaviour and preferences has become essential for maintaining a competitive edge. As we enter 2024, the significance of retail customer analytics is increasingly evident. This comprehensive guide delves into the realm of retail customer analytics, its pivotal role in contemporary business strategies, key applications, challenges, implementation methodologies, and the future trajectory of customer analytics.
What is Retail Customer Analytics?
Retail customer analytics encompasses the systematic collection, analysis, and interpretation of customer behaviour and preference data. This data-driven approach enables retailers to gain profound insights into customer dynamics, optimize marketing strategies, and enhance the shopping experience.
Key data sources utilized in retail customer analytics include:
- Point of Sale Transactions: These provide granular insights into product purchases, purchase frequency, and customer buying patterns, forming the foundation for analysing sales trends and customer preferences.
- Website Clicks and Purchases: E-commerce platforms generate data on user interactions, revealing navigation patterns, product interests, and purchase drivers.
- Browsing and Shopping Cart Behaviour: Analysing customer browsing patterns and shopping cart data helps identify potential friction points, such as pricing issues or checkout process inefficiencies.
- Loyalty Program Data: Loyalty programs offer longitudinal views of customer interactions and preferences, aiding in the identification of loyal customers and the design of targeted promotions.
- Product Reviews and Ratings: Customer feedback through reviews and ratings provides direct insights into product performance, helping identify popular items and areas for improvement.
- Social Media Activity: Data from social media platforms reveals customer sentiment, emerging trends, and brand perception, enabling real-time engagement and trend tracking.
- Email Engagement: Metrics such as open rates, click-through rates, and conversion rates provide insights into the effectiveness of email marketing strategies and help refine campaign approaches.
Why Retail Customer Analytics is Critical
Retail customer analytics is crucial for several reasons:
- Enhanced Customer Understanding: Provides a deeper comprehension of customer preferences and behaviours, allowing for more tailored offerings.
- Optimized Marketing Efforts: Identifies the most effective marketing channels and messages, improving campaign efficiency and ROI.
- Improved Customer Experience: Enables personalized interactions and enhanced service delivery.
- Informed Decision-Making: Offers actionable insights into market trends and business performance, supporting strategic decisions.
Critical Applications of Retail Customer Analytics
- Marketing Campaign Optimization: Analytics helps identify high-impact marketing campaigns by assessing performance across channels, enabling resource allocation to the most effective strategies and improving ROI.
- Inventory Management: By understanding demand patterns, analytics aids in optimizing inventory levels, ensuring popular products are available while minimizing excess stock.
- Personalization: Enables the creation of customized shopping experiences through tailored product recommendations, promotions, and communications based on individual customer data.
- Pricing Strategies: Informs dynamic pricing strategies by analyzing customer responses to price changes and competitive pricing, aligning prices with market demand.
- Customer Segmentation: Allows for the segmentation of customers based on behaviour and preferences, facilitating targeted marketing and enhanced engagement.
- Customer Retention: Identifies factors influencing customer retention and churn, enabling the implementation of strategies such as loyalty programs and personalized offers to boost retention.
Overcoming Challenges in Retail Customer Analytics
- Data Quality and Integration: Maintaining high data quality across diverse sources requires robust data integration and cleansing processes to ensure accuracy and consistency.
- Data Privacy and Security: Compliance with data protection regulations and the implementation of stringent security measures are essential to safeguard customer data and address privacy concerns.
- Skilled Workforce: Building a team with the expertise to analyze and interpret data is crucial. Investment in training and development is necessary to cultivate a skilled analytics workforce.
- Technology Infrastructure: A robust technology infrastructure is required to manage large data volumes and perform complex analyses. Investing in advanced analytics tools and platforms supports effective data handling.
How to Effectively Implement Retail Customer Analytics
- Define Objectives: Establish clear objectives for analytics initiatives, such as improving customer satisfaction or optimizing marketing strategies.
- Identify Key Metrics: Determine the key performance indicators (KPIs) aligned with your objectives, including sales data, customer satisfaction scores, and conversion rates.
- Collect Data: Aggregate data from various sources such as POS systems, e-commerce platforms, and social media, ensuring data accuracy and consistency.
- Analyse Data: Utilize advanced analytics techniques to analyze the data. Employ descriptive analytics for historical insights, predictive analytics for forecasting, and prescriptive analytics for actionable recommendations.
- Derive Insights: Extract actionable insights from the analysis, identifying patterns, trends, and correlations to inform business decisions.
- Implement Actions: Execute strategies based on the insights, including new marketing campaigns, pricing adjustments, or inventory optimizations.
- Monitor and Adjust: Continuously evaluate the performance of implemented actions and adjust strategies as needed to maintain alignment with business goals.
The Future with Customer Analytics
The future of retail customer analytics is set to be shaped by several emerging trends:
- Artificial Intelligence and Machine Learning: AI and ML are enhancing analytics capabilities by enabling more sophisticated data analysis and prediction, uncovering patterns that traditional methods may miss.
- Real-Time Analytics: Enables immediate decision-making based on current data, facilitating dynamic pricing, real-time inventory management, and responsive marketing.
- Enhanced Personalization: Advances in analytics will drive deeper personalization, offering highly customized shopping experiences and targeted promotions.
- Integration with IoT: IoT devices generate vast amounts of data, and integrating this with customer analytics provides richer insights into customer behaviour and preferences.
- Ethical Considerations: As analytics capabilities grow, addressing data privacy, security, and transparency will be crucial for maintaining customer trust and ensuring ethical data practices.
Conclusion
Retail customer analytics is a potent tool for unlocking business potential. By harnessing data from diverse sources, retailers can gain a comprehensive understanding of customer behaviour, optimize marketing strategies, and enhance the shopping experience. Despite the challenges, the benefits of retail customer analytics are substantial. With ongoing technological advancements, the future of customer analytics holds promising opportunities for retailers to excel in a competitive market. Effective implementation and utilization of customer analytics can position businesses for long-term success and growth.
FAQ
What is retail customer analytics, and why is it important?
Retail customer analytics involves the systematic collection and analysis of data related to customer behaviour and preferences. It is vital because it helps retailers understand their customers better, tailor their offerings, optimize marketing strategies, and enhance the overall shopping experience. This data-driven approach supports strategic decision-making and helps businesses remain competitive in the evolving retail environment.
What types of data are typically used in retail customer analytics?
Retail customer analytics utilizes various data sources, including:
- Point of Sale (POS) Transactions: Data on product purchases and customer buying patterns.
- Website Clicks and Purchases: Insights into online navigation and purchase behaviours.
- Browsing and Shopping Cart Behaviour: Data on products considered but not purchased.
- Loyalty Program Data: Longitudinal data on customer behaviour and preferences.
- Product Reviews and Ratings: Direct feedback on product performance.
- Social Media Activity: Data on customer sentiment and brand perception.
- Email Engagement: Metrics on email open rates, click-through rates, and conversions.
How can retail customer analytics improve marketing campaigns?
Retail customer analytics enhances marketing campaigns by identifying the most effective channels, messages, and strategies. Through data analysis, retailers can:
- Design targeted campaigns for specific customer segments.
- Allocate resources more efficiently to high-impact marketing activities.
- Improve ROI by focusing on strategies that drive engagement and sales.
- Personalize marketing messages to enhance customer satisfaction and loyalty.
What are some challenges associated with implementing retail customer analytics?
Challenges in implementing retail customer analytics include:
- Data Quality and Integration: Ensuring data accuracy and consistency across various sources.
- Data Privacy and Security: Complying with regulations and protecting customer data.
- Skilled Workforce: Developing a team with the expertise to analyze and interpret data.
- Technology Infrastructure: Investing in advanced tools and platforms to manage data and perform analyses.
What trends are shaping the future of retail customer analytics?
Key trends shaping the future of retail customer analytics include:
- Artificial Intelligence and Machine Learning: Facilitating advanced data analysis and predictive capabilities.
- Real-Time Analytics: Enabling immediate, data-driven decision-making.
- Enhanced Personalization: Offering more customized shopping experiences.
- Integration with IoT: Providing deeper insights through data from connected devices.
- Ethical Considerations: Addressing data privacy and transparency to maintain customer trust.